Mado Ntekouli was trained as a computer engineer in her home country Greece, and via a masters programme in Delft, she ended up in Maastricht to do a PhD within the New Science of Mental Disorders consortium. Where does she stand after 1,5 years of her PhD?
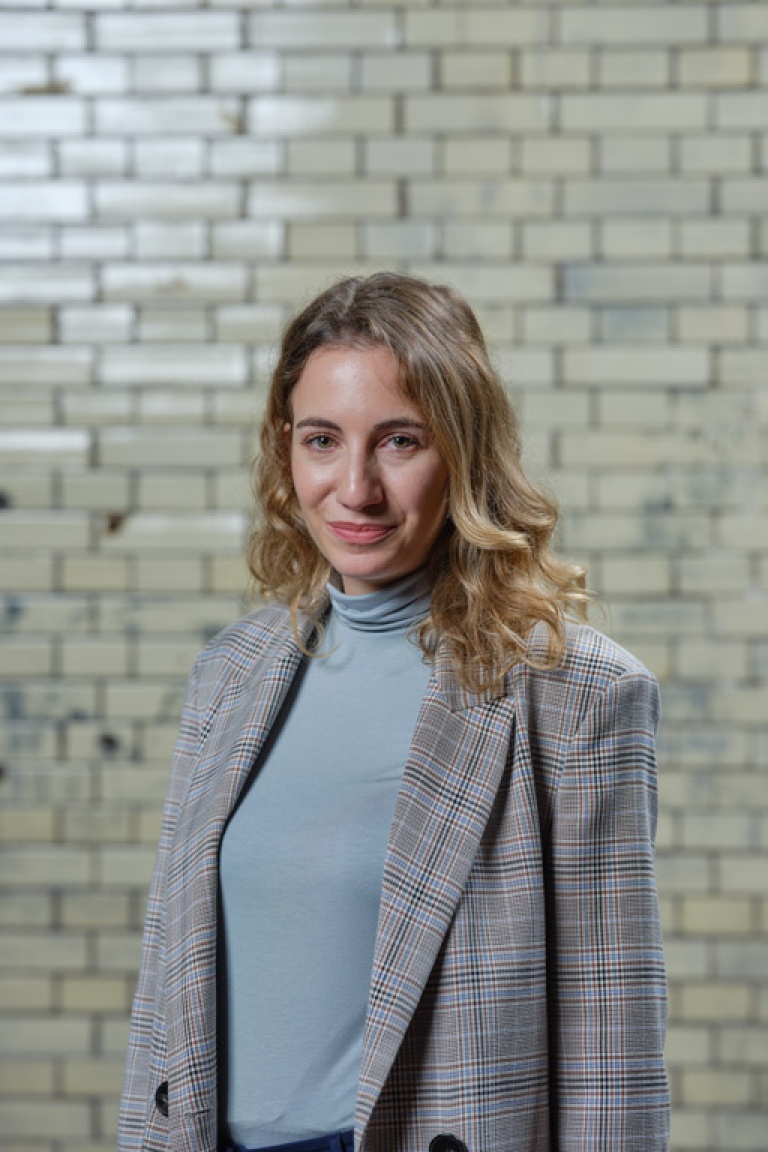
As a biomedical computer engineer, you could probably find a job with a large salary in industry. Why did you choose scientific research?
“During my bachelor’s programme Electrical and Computer Engineering in Greece I first experienced what doing research means. I like the whole process of searching, getting results, struggling with challenges and then the excitement when you find something and the experts say: ‘That’s a publishable outcome’. It’s very rewarding to me. My bachelor’s thesis was in the field of biomedical informatics. It was published at a conference, that stimulated my interest in research too. Since then, my goal is to solve medical problems, using my engineering tools.”
And why did you choose the Netherlands for your master’s and PhD?
“Biomedical Engineering, the master’s programme that fitted my interests best, is not so popular in Greece yet, so I looked for a good university abroad and ended up in Delft. The first year was very intense, with courses on anatomy and physiology that were all new to me, but in the end it was a good experience. My master’s thesis was on techniques used for visualizing brain activity. I looked for a PhD position in The Netherlands, or close by, because I like this country. I don’t exactly know why it almost feels like home here. In Delft, there was quite a large Greek student community, and here in Maastricht, my supervisor Jerry Spanakis is Greek as well. But I like the relaxed way of the Dutch too.”
So can you explain what you worked on these first 1,5 years of your project?
“The focus of my research is on the predictive network analysis of large-scale, dynamically interacting variables, such as symptoms, collected from multimodal data sources. So I’m looking for a computational model that is capable of capturing and predicting human behaviour. An accurate model is important for exploring data, like our consortium will be doing. We presume that the interaction between two variables, like ‘I feel unhappy’ and ‘I eat unhealthy food’ is not a straight, linear line. These more complex patterns can be captured in more advanced, non-linear models and we tried to apply some existing models on large, complex data sets, both real data and synthetic data. We found with great consistency that the non-linear models outperform the linear models. While this was all on data of individuals, we then checked if aggregated data from a group could outperform this non-linear model in predicting probabilities of human behaviour. For example: can you predict if an individual will eat unhealthy within two hours, based on the data? I found that the ‘Knowledge Distillation’ model could give promising results and it can outperform in many cases even the non-linear model, but of course the results changed a lot across participants. So that depends on the data, the number of time points we have for each participant and that leads to varying results, but in general it’s promising.”
These models you work with, already exist, or did you develop them yourself?
“The Knowledge Distillation method is well known in neural networks, but I tried to adjust it a bit to my non-linear model and the large numbers of data we want to work with. We have hundreds of participants in the end in our NSMD studies. Of course, each participant is unique, but more participants can give some complementary insights.”
You wrote down your findings in a paper that was accepted at a conference?
“Yes, the paper was accepted by the Intelligent Data Analysis Conference in Rennes, France. I presented it there in April this year. Very happy with that.”
What’s the next challenge you’ll work on?
“That’s the idea of clustering participants that are more similar. It’s called the time series clustering problem and the challenge is: you don’t know if the people you select are indeed similar. There are many different distance metrics to find how similar participants are. If I find that the information of all can be used in a complementary way in models, it can give better results at the individual level as well.”
When are you most happy in your work?
“When I find interesting results, which I can elaborate and improve my models on. And when I read something in the literature that’s interesting, a method that we may be able to apply to EMA data (Ecological Momentary Assessment; the repeated sampling of subjects' current behaviours and experiences in real time, in subjects' natural environments, ed.). There are many different models out there and we want to find what best applies to our many participants and the many data we get. I’m also very interested in incorporating the data we get from smartphones during studies, for example on social media usage or sleep. They are more objective data that can add value to the EMA data. That’s promising, I think.”